THIS PAGE PERTAINS TO LAST YEAR’S EVENT.
Sign up for our DLW Updates to receive the latest news on the 2020 event.
Review – Agenda 2019
Deep Learning World Las Vegas 2019
June 16-20, 2019 – Caesars Palace, Las Vegas
This page shows the agenda for Deep Learning World. Click here to view the full 7-track agenda for the five co-located conferences at Mega-PAW (PAW Business, PAW Financial, PAW Healthcare, PAW Industry 4.0, and Deep Learning World).
Workshops - Monday, June 17th, 2019
Full-day: 8:30am – 4:30pm
This one-day introductory workshop dives deep. You will explore deep neural classification, LSTM time series analysis, convolutional image classification, advanced data clustering, bandit algorithms, and reinforcement learning. Click workshop title above for the fully detailed description. Click workshop title above for the fully detailed description.
The Mega-PAW event of which Deep Learning World is a part also offers additional analytics workshops which do not pertain to deep learning, but cover other analytics topics.
Click here for the full list of workshops.
Day 1 - Tuesday, June 18th, 2019
A veteran applying deep learning at the likes of Apple, Samsung, Bosch, GE, and Stanford, Mohammad Shokoohi-Yekta kicks off Mega-PAW 2019 by addressing these Big Questions about deep learning and where it's headed:
- Late-breaking developments applying deep learning in retail, financial services, healthcare, IoT, and autonomous and semi-autonomous vehicles
- Why time series data is The New Big Data and how deep learning leverages this booming, fundamental source of data
- What's coming next and whether deep learning is destined to replace traditional machine learning methods and render them outdated
In the United States, between 1500 and 3000 infants and children die due to abuse and neglect each year. Children age 0-3 years are at the greatest risk. The children who survive abuse, neglect and chronic adversity in early childhood often suffer a lifetime of well-documented physical, mental, educational, and social health problems. The cost of child maltreatment to American society is estimated at $124 - 585 billion annually.
A distinctive characteristic of the infants and young children most vulnerable to maltreatment is their lack of visibility to the professionals. Indeed, approximately half of infants and children who die from child maltreatment are not known to child protection agencies before their deaths occur.
Early detection and intervention may reduce the severity and frequency of outcomes associated with child maltreatment, including death.
In this talk, Dr. Daley will discuss the work of the nonprofit, Predict-Align-Prevent, which implements geospatial machine learning to predict the location of child maltreatment events, strategic planning to optimize the spatial allocation of prevention resources, and longitudinal measurements of population health and safety metrics to determine the effectiveness of prevention programming. Her goal is to discover the combination of prevention services, supports, and infrastructure that reliably prevents child abuse and neglect.
The research on the state of Big Data and Data Science can be truly alarming. According to a 2019 NewVantage survey, 77% of businesses report that "business adoption” of big data and AI initiatives are a challenge. A 2019 Gartner report showed that 80% of AI projects will “remain alchemy, run by wizards” through 2020. Gartner also said in 2018 that nearly 85% of big data projects fail. With all these reports of failure, how can a business truly gain insights from big data? How can you ensure your investment in data science and predictive analytics will yield a return? Join Dr. Ryohei Fujimaki, CEO and Founder of data science automation leader dotData, to see how Automation is set to change the world of data science and big data. In this keynote session, Dr. Fujimaki will discuss the impact of Artificial Intelligence and Machine Learning on the field of data science automation. Learn about the four pillars of data science automation: Acceleration, Democratization, Augmentation and Operationalization, and how you can leverage these to create impactful data science projects that yield results for your business units and provide measurable value from your data science investment.
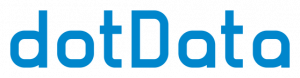
Over the last few years, convolutional neural networks (CNN) have risen in popularity, especially in the area of computer vision. Many mobile applications running on smartphones and wearable devices would potentially benefit from the new opportunities enabled by deep learning techniques. However, CNNs are by nature computationally and memory intensive, making them challenging to deploy on a mobile device. We explain how to practically bring the power of convolutional neural networks and deep learning to memory and power-constrained devices like smartphones. We’ll illustrate the value of these concepts with real-time demos as well as case studies from Google, Microsoft, Facebook and more. You will walk away with various strategies to circumvent obstacles and build mobile-friendly shallow CNN architectures that significantly reduce memory footprint.
Deep learning certainly has roots in the autonomous vehicle space. However, most trucking companies have a substantial investment in existing class 8 semi-trailer trucks that are not going to be replaced overnight. Trimble Transportation Mobility is using deep learning technologies, in conjunction with other advanced analytic techniques and state of the art DevOps approaches to help ensure the safe operation of trucking fleets. While it may be premature for many trucking fleets to embrace autonomous vehicles, TTM has made it possible for those same companies to leverage deep learning as a way to reduce costs and improve safety.
Field issue (malfunction) incidents are costly for the manufacturer’s service department. A normal telematics system has difficulty in capturing useful information even with pre-set triggers. In this session, Yong Sun will discuss how a machine learning, deep learning based predictive software/hardware system has been implemented to solve these challenges by 1) identifying when a fault will happen 2) diagnosing the root cause on the spot based on time series data analysis. Yong Sun will cover a novel technique for addressing a lack of training data for the neural network based root cause analysis.
Your advanced analytics efforts aren’t all that in synch with your overall business strategy. Don’t fret – it seemingly happens to everyone. How do you spot it? What should you do about it? In this session, we’ll briefly address how to spot the issue and a few techniques to achieve much improved alignment. Hint, we may suggest that’s it’s a bit crazy to try and find a single person with knowledge and expertise in math, statistics, programming, data wrangling, modeling AND exceptional business knowledge!
Machine learning has been sweeping our industry, and the creativity it is already enabling is incredible. On the flip side there has also been the emergence of technology like Deep Fakes with the possibility to spread disinformation. As a tool maker, is our technology neutral, or are we responsible for creating technology for good? How should we be thinking about biases of multiple forms when training AI? What can go wrong when learning is applied to indiscriminate user data?
At Adobe we look at this problem from multiple angles, from weighing the positives of technology against their possible misuses, researching detection technology for manipulated images, assembling diverse teams of experts, and having internal training and reviews of technology around Artificial Intelligence.
At PayPal achieving four nines of availability is the norm. In the pursuit of exponentially complex additional nines we have recently embarked on applying deep learning to forecasting datacenter metrics. With little shared to the open community, this talk will shine light on how we apply Seq2Seq networks to forecasting CPU and Memory metrics at scale. In sharing ideas around building deep networks for forecasting; the talk will highlight how life of Data Scientists at PayPal has been greatly simplified by the use of Template Notebooks stitched into stateful and stateless pipelines using PayPal’s open source PPExtensions.
How much data is enough to build an accurate deep learning model? This one of the first and most difficult questions to answer early in any machine learning project. However, the quality and applicability of your data are more important considerations than quantity alone. This talk presents some insights and lessons learned for gauging the suitability of electronic health record (EHR) training data for a life underwriting project. You will see how to determine if more data might increase accuracy and how to identify any weaknesses a deep neural network might have as a result of your current training data.
There have been ten US recessions since 1950. When is the next one? The answer matters because asset prices plunge in recessions, which creates both risk and opportunity. Forecasters answer this question by looking at leading economic indicators. We translate the thinking of forecasters into machine learning solutions. This talk explains the use of recurrent neural networks, which excel at learning historical patterns that don’t repeat, but rhyme. Our model anticipates the Great Recession from past data and exhibits lower error than established benchmarks. The proposed approach is broadly applicable to other prediction problems such as revenue and P&L forecasting.

Day 2 - Wednesday, June 19th, 2019
Deep neural networks provide state-of-the-art results in almost all image classification and retrieval tasks. This session will focus on the latest research on active learning and similarity search for deep neural networks and how they are applied in practice by the Verizon Media Group. Using active learning, we can select better images and substantially reduce the number of images required to train a model. It enables us to achieve state-of the art performance while substantially reducing cost and labor. By using triplet loss for similarity search, we can improve our ability to retrieve better images for shopping application and advertising.
An introduction to the basics of Neural Networks (NN) in the Wolfram language and how NN layers can be connected into either Chains or Graphs to construct Deep Learning networks. We will cover basic constructs like optimization through Stochastic Gradient Descent, Encoders and Decoders, NN Layers of different types, Containers for the layers, the problem of overfitting, and examples from Wolfram Neural Net Repository. References include http://reference.wolfram.com/language/guide/NeuralNetworks.html and http://resources.wolframcloud.com/NeuralNetRepository/
On the forefront of deep learning research is a technique called reinforcement learning, which bridges the gap between academic deep learning problems and ways in which learning occurs in nature in weakly supervised environments. This technique is heavily used when researching areas like learning how to walk, chase prey, navigate complex environments, and even play Go. In this session, Martin Görner will detail how a neural network can be taught to play the video game Pong from just the pixels on the screen. No rules, no strategy coaching, and no PhD required. Martin will build on this application to show how the approach can be generalized to other problems involving a non-differentiable steps that cannot be trained using traditional supervised learning techniques.
This is a prelude to Martin’s full day workshop on Thursday, June 20th: Hands-On Deep Learning in the Cloud: Fast and Lean Data Science with Tensorflow, Keras, and TPUs.
Application of advanced analytics techniques like machine learning and deep learning is in the initial stages in airline industry. In this presentation, we outline how this technology is being applied and GE Aviation's experience in advancing this for the last 7 years.
In this talk, Chandra Kahtri, Senior AI Scientist at Uber AI, formerly at Alexa AI, will detail various problems associated with Conversational AI such as speech recognition, language understanding, dialog management, language generation, sensitive content detection and evaluation and the advancements brought by deep learning in addressing each one of these problems. He will also present on the applied research work he has done at Alexa and Uber for the problems mentioned above.
In applications like fraud and abuse protection, it is imperative to use progressive learning and fast retraining to combat emerging fraud vectors. However, somewhat unfortunately, these scenarios also suffer from the problem of late-coming supervision (such as late chargebacks), which makes the problem even more challenging! If we use a direct supervised approach, a lot of the valuable sparse supervision signal gets wasted on figuring out the manifold structure of data before the model actually starts discriminating newly emerging fraud. At Microsoft we are investigating unsupervised learning, especially auto encoding with deep networks, as a preprocessor that can help tackle this problem. An auto-encoding network, which is trained to reconstruct (in some sense) the input features through a constriction, learns to encode the manifold structure of the data into a small set of latent variables, similar to how PCA encodes the dominant linear eigen spaces. The key point is that the training of this auto-encoder happens with the abundant unlabeled data – it does not need any supervision. Once trained, we then use the auto-encoder as a featurizer that feeds into the supervised model proper. Because the manifold structure is already encoded in the auto-encoded bits, the supervised model can immediately start learning to discriminate between good and bad manifolds using the precious training signal that flows in about newly emerging fraud patterns. This effectively improves the temporal tracking capability of the fraud protection system and significantly reduces fraud losses. We will share some promising early results we have achieved by using this approach.
Logs are a valuable source of data, but extracting knowledge is not easy. To get actionable information, it frequently requires creating dedicated parsing rules, which leaves the long-tail of less popular formats. Widely applying real-time pattern discovery establishes each log as its own event of a given type (pattern) with specific properties (parameters). This application makes it a tremendous input source for Deep Learning algorithms that filter out noise and present what’s most interesting. This talk reviews real-life cases where these techniques allowed to pinpoint important issues, and highlights insights on how best to elevate DL in the development lifecycle.
Automated modeling is already in focus by practitioners. However, applications for marketing campaigns require significant effort in data preparation. To address this bottleneck, the robotic modeler integrates a front layer, which automatically scrolls executed campaigns and prepares data for modeling, with a machine learning engine. It enables for automated campaign backend modeling, generates scoring codes, and produces supportive documentations. The robotic modeler supports generalized deep learning assembling business targets and features. Systematically running the robotic modeler provides additional benefits including perceiving input feature importance from various campaigns or estimating cross-campaign effects. It empowers “hyper-learning” derived from campaign modeling.
Deep learning models have shown great success in commercial applications such as self driving cars, facial recognition and speech understanding. However, typically these models require a large amount of labeled data, presenting significant hurdles for AI startups faced with a lack of data, funding and resources. In this session, I will discuss how to overcome the cold-start problem of deep learning by using transfer learning, synthetic data generation, data augmentation and active learning. This talk will go through a real use case of invoice processing and information extraction, which is a critical step in the Account Payable process.
In the talk, I will give a detailed example how a seamlessly integrated, distributed Spark + Deep Learning system can reduce training cost by 90% and increase prediction throughput by 10X. With such a powerful tool in hand, a data scientist can process more data and get more data insight than a team of 20 data scientists with traditional tools.
Workshops - Thursday, June 20th, 2019
Full-day: 8:30am – 4:30pm
During this workshop, you will gain hands-on experience deploying deep learning on Google’s TPUs (Tensor Processing Units) – held the day immediately after the Deep Learning World and Predictive Analytics World two-day conferences.. Click workshop title above for the fully detailed description.
The Mega-PAW event of which Deep Learning World is a part also offers additional analytics workshops do not pertain to deep learning, but cover other analytics topics.
Click here for the full list of workshops.