Deep Learning World Las Vegas 2020
May 31-June 4, 2020 – Caesars Palace, Las Vegas
This page shows the agenda for Deep Learning World. Click here to view the full 7-track agenda for the five co-located conferences at Mega-PAW (PAW Business, PAW Financial, PAW Healthcare, PAW Industry 4.0, and Deep Learning World).
TRACK TOPICS – The two tracks of the main two-day conference cover these topics:
TRACK TOPICS – The two tracks of the main two-day conference cover these topics:
Deep Learning World - Las Vegas - Day 1 - Tuesday, June 2nd, 2020
A veteran applying deep learning at the likes of Apple, Bosch, GE, Microsoft, Samsung, and Stanford, Mohammad Shokoohi-Yekta kicks off Machine Learning Week 2020 by addressing these Big Questions about deep learning and where it's headed:
- Late-breaking developments applying deep learning in retail, financial services, healthcare, IoT, and autonomous and semi-autonomous vehicles
- Why time series data is The New Big Data and how deep learning leverages this booming, fundamental source of data
- What's coming next and whether deep learning is destined to replace traditional machine learning methods and render them outdated
As principles purporting to guide the ethical development of Artificial Intelligence proliferate, there are questions on what they actually mean in practice. How are they interpreted? How are they applied? How can engineers and product managers be expected to grapple with questions that have puzzled philosophers since the dawn of civilization, like how to create more equitable and fair outcomes for everyone, and how to understand the impact on society of tools and technologies that haven't even been created yet. To help us understand how Google is wrestling with these questions and more, Jen Gennai, Head of Responsible Innovation at Google, will run through past, present and future learnings and challenges related to the creation and adoption of Google's AI Principles.
As the economy continues its uncertain path, businesses have to expand reliance on data to make sound decisions that directly impact the business - from managing cash flow to planning product promotion strategies, the use of data is at the heart of mitigating the risks of a recession as well as planning for a recovery. Predictive Analytics, powered by Artificial Intelligence (AI) & Machine Learning (ML), has always been at the forefront of using data for planning. Still, most companies struggle with the techniques, tools, and with lack of resources needed to develop and deploy predictive analytics in meaningful ways. Join dotData CEO, Ryohei Fujimaki to learn how automation can help Business Intelligence teams develop and add AI and ML-powered technologies to their BI stack through AutoML 2.0, and how organizations of all sizes can solve the predictive analytics challenge in just days without adding additional resources or expertise.
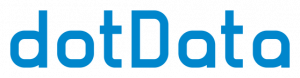
At Standard Cognition we are solving the problem of automated scene understanding for cashierless checkout. Building and maintaining machine learning systems that can be deployed to hundreds of stores poses many machine learning and engineering challenges.
While many canonical problems in the deep learning literature have focused on solutions that are comprised of a single network or an ensemble of networks for a fixed dataset, a production system may have multiple, modular models that cascade into each other and are trained and evaluated on moving datasets. Such systems of modular deep neural networks have advantages in sample complexity, development scalability through division of labor, and deployment scalability through reusable and testable intermediate outputs, but come at the cost of managing additional complexity.
Through ensuring reproducible and shareable data-dependent state, we improve our ability to make continuous progress in arbitrarily complex multi-model systems, especially as Standard Cognition scales in number of developers and amount of data.
For 6sense’s machine learning models, a person’s level and function is an important feature in predicting if a lead will move to an open opportunity and/or closed won. Often, only a job title is available. A human can deduce their associated level and function relatively easily but becomes a large effort at scale. With millions of jobs needing classification on a daily basis, we moved from a rules-based SQL string-matching method to utilizing LSTM neural networks. Our implementation of these deep learning models can now classify a job title’s level and function with greater accuracy, speed, and usability.
Genesys PureCloud supports ~100k users making over 60M API calls every day and the volume of data requires an automated system to detect "insider threat" based on user behavior. Because there are few if any examples of this behavior, we developed an anomaly detection system based on deep-learning: in particular, using Transformer networks to learn the probability of a given API call based on a sequence of previous API calls. We compare the detection capability with simpler models (such as Markov chains) and show how anomaly detection can give real-time threat prediction.
Ari Kaplan will talk about his real-life Moneyball experiences of disruption in Major League Baseball front offices - and how artificial intelligence will disrupt every business industry. Having helped lead the adoption of data science throughout baseball, including creating the Chicago Cubs analytics department, he will lead lively discussion on how winning in baseball translates to winning across other industries, overcoming cultural resistance, and doing analytics at scale and velocity to win the race.

Data Science in general and Deep Learning in particular continue to reshape the future of the Energy sector across various segments. From exploration, development and production to downstream and new energies business, measurable value of digitalization has been observed in both efficiencies and savings. Deep Learning is one of the key underlying enablers for creating competitive advantage. This presentation provides an overview of some of use case applications and lessons learned from establishing a platform that progress ideas to embedded business enablers.
Large scale distributed training has become an essential element to scaling the productivity for ML engineers. Today, ML models are getting larger and more complex in terms of compute and memory requirements. The amount of data we train on at Facebook is huge. This talk outlines distributed training platform that is used in large scale ranking models across Facebook. In this talk, we will learn about the distributed training platform to support large scale data and model parallelism. The talk will also touch base on how this platform is used to express large scale models (Ads ranking, news feed ranking, search, etc), the system used to train this model, and production considerations. You will also learn about the distributed training support for PyTorch and how we are offering a flexible training platform for ML engineers to increase their productivity at facebook scale.
Road traffic crashes have reached epidemic proportions with 1.35 million deaths and over 50 million people injured in 2018. It’s no surprise that, according to internal company data, 70 percent of severe collisions involve a distracted driver. That means, in the midst of developing driverless cars for the distant future, we must prioritize keeping drivers and pedestrians safe today. The first step: reduce distracted driving and re-train drivers to focus on the road.
To make roads safer, it’s imperative to have technology that is proven to help fleets and commercial drivers across the globe. Nauto, the only company with real-time AI-powered Driver Behavior Learning Platform that helps predict and prevent high-risk driving events, has created technology to improve and change driver behavior and reduce distracted driving. Nauto’s AI analyzes billions of data points from millions of miles driven to provide accurate insights to improve driver safety.
In this session, Shweta Shrivastava and Piyush Chandra will dive into how automakers and fleets can utilize the power of AI on the edge to better assess risky driver behavior and provide real-time solutions for drivers while creating safer roads. They will also explore the best practices organizations can take when building and training AI models for driver safety initiatives.
US is affected by severe natural disasters annually. It is important to estimate the damage quickly in order to respond with adequate measures. Current analytical bottleneck occurs due to manual review of the post-disaster areal imagery. Our goal was to develop an algorithm to detect damaged buildings in satellite images.
We used semantic segmentation techniques to train custom models for buildings detection and further damage assessment. We have split the problem into building localization and roof damage detection. Custom roof damage dataset has been created containing 3,000+ images from hurricane Michael satellite images.
The vast majority of machine learning is supervised learning. Supervised learning can tell us what will happen, but it can't tell us what to do. Let's call that area of machine learning "reasoning", and it compliments supervised learning to power some of the world's most popular websites. Deep reinforcement learning has shown to be incredibly promising reasoning tool.
This talk will cover a variety of approaches to reasoning, including hand-written rules, black box optimization, multi-armed bandits, and deep reinforcement learning. The talk will also introduce ReAgent, an end-to-end open source platform for reasoning and deep RL, and how Facebook is using it for growth campaigns, ad coupons, infrastructure optimization and novel approaches to recommendations ranking.
Deep Learning World - Las Vegas - Day 2 - Wednesday, June 3rd, 2020
Marketing leads are exposed numerous channels, creating complex cross-channel relationships and making effectiveness of the campaign difficult to comprehend and execute. Are there some path-sequences that are better at driving leads than others? To answer this question, we at The Vanguard Group build Bayesian based RNN models and conduct path analysis for marketing campaigns. This presentation will detail how the Bayesian approach to RNN models makes them robust in the presence of noise and uncertainties. We'll go on to show how we interpret the results and make them actionable by visualizing the latent space and performing ‘Next best Action’ on the potential leads, maximizing the impact of channel treatments towards any desired outcome.
Until a machine learning model is in production, it’s a cost center for a business, consuming time, skilled people, money, and equipment. When machine learning models go into operational systems, that turns around. Projects show powerful business value. But that final hurdle is the point of failure for far too many projects. Even then, the job isn’t done. The need to continually score, manage, and update with new models never stops.
Learn how to rapidly get deep learning models hooked into operational data architectures with PMML, and manage models as an integral part of the process.
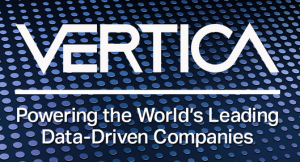
Generative approaches enable creating numerous scenarios coherent with the reality, as long as the representations are good approximations of the real world. In this talk, I will discuss my work at Purdue, DeepScale, and Facebook in extracting such generative representations from 2D and 3D data for mapping, modeling, and reconstruction of spatial data and urban models; combining computer vision, machine learning, and computational geometry for shape understanding. In the second part of the talk, I will introduce FakeCatcher, a unique system that detects deep fake videos in the wild, with high accuracy. I will conclude by showcasing how generative approaches can be utilized in our volumetric capture stage at Intel Studios.
The session will cover some applications and use-cases of Deep Learning as applicable to large-scale payments fraud detection. Such e-commerce is inherently heterogeneous with multi-objective criteria replete with diverse and ever-evolving fraud patterns, as also in need of quick transaction adjudication timelines to enhance customer experience. Furthermore, traditional machine learning as applicable to such problems involves domain-centric assumptions, as also manually engineered feature spaces with redundancy and correlation. The talk will elaborate upon multiple problem formulations involving deep learning methods for applications such as robust temporal representation learning and multi-task learning across different sub-populations. Methods based on generative modeling with multiple objectives such as reducing the decline of good users while sustaining a high fraud catch rate will be also be covered
Anomaly detection has many applications, such as intrusion detection, fraud detection, video surveillance, and IoT sensor data. Deep Anomaly Detection (DAD) performs exceptionally well on image data and sequential data, and it is essential for feature extraction when the data scale is large, i.e., over a terabyte. Le Zhang investigates various traditional and deep anomaly detection algorithms and their applications at Walmart. Le has applied anomaly detection techniques to many business problems, including fraud detection, abnormal spending detection, and irregular demand identification. In this session, you will learn different anomaly detection techniques and their performance on various datasets.
A major drawback of employing DNNs in practical settings is that with millions of parameters to be trained they require access to a massive amount of data. We use a state-of-the-art technique called Cross-language Transfer Leaning to alleviate the cold-start problem in NLP DL models for predicting best shipping in emerging markets. Although the language, the prediction tasks and the network architectures are quite different across markets, we exhibit how we can utilize the learned features in one network to significantly improve the accuracy score in the target network/market.
Recent success of sequence based deep learning models on NLP tasks have drawn attention to their application in time series data analysis. At Verizon, we are exploring RNN, LSTM models to solve our business problems involving time series data. Verizon has rich time series data sets on network cell site performance, customer experience and geospatial information. In my talk, I will discuss how we are analyzing these datasets using deep learning techniques to:
- Improve nationwide customer experience
- Improve network performance
- Optimize capital allocation
Many organizations respond to inquiries, whether internal or external, over text chats or support tickets. Frequently, the answers to these questions can be found in knowledge bases. We’ll discuss how we at Google approached automatically suggesting the most relevant knowledge base articles using dual encoders and deep learning-based natural language processing. We’ll talk through how this fits into the machine learning project lifecycle, with examples of common pitfalls.
The talk covers use cases, special challenges and solutions for building Interpretable and Secure AI systems using Pytorch.
You will learn about:
- Tools for building Interpretable models
- How to build secure, privacy preserving AI models with Pytorch?
- Use cases and insights from the field