Review – Agenda 2021
Deep Learning World 2021
May 24-28, 2021 – Livestreamed
To view the full 7-track agenda for the six co-located conferences at Machine Learning Week click here or for the individual conference agendas here: PAW Business, PAW Financial, PAW Healthcare, PAW Industry 4.0, PAW Climate or Deep Learning World.
Workshops - Wednesday, May 19th, 2021
Full-day: 7:15am – 2:30pm PDT
This one-day session surveys standard and advanced methods for predictive modeling (aka machine learning).
Full-day: 7:30am – 3:30pm PDT
Gain experience driving R for predictive modeling across real examples and data sets. Survey the pertinent modeling packages.
Full-day: 8:00am – 3:00pm PDT
This one day workshop reviews major big data success stories that have transformed businesses and created new markets.
Workshops - Thursday, May 20th, 2021
Full-day: 8:00am – 3:00pm PDT
This workshop dives into the key ensemble approaches, including Bagging, Random Forests, and Stochastic Gradient Boosting.
Full-day: 8:00am – 3:00pm PDT
Python leads as a top machine learning solution – thanks largely to its extensive battery of powerful open source machine learning libraries. It’s also one of the most important, powerful programming languages in general.
Full-day: 8:00am – 3:00pm PDT
Machine learning improves operations only when its predictive models are deployed, integrated and acted upon – that is, only when you operationalize it.
Full-day: 8:30am – 3:30pm PDT
Gain the power to extract signals from big data on your own, without relying on data engineers and Hadoop specialists.
Workshops - Friday, May 21st, 2021
Full-day: 7:15am – 2:30pm PDT
This one-day session reveals the subtle mistakes analytics practitioners often make when facing a new challenge (the “deadly dozen”), and clearly explains the advanced methods seasoned experts use to avoid those pitfalls and build accurate and reliable models.
Deep Learning World - Virtual - Day 1 - Monday, May 24th, 2021
Amazon's vision is to be earth's most customer-centric company. This talk explores how the Alexa Hybrid Science team in Pittsburgh, PA applies a customer-centric lens to cutting-edge machine learning research. The team is responsible for developing on-device Alexa automatic speech recognition models to provide a faster, more reliable Alexa experience. Our research includes neural network compression techniques, end-to-end spoken language understanding and optimizing machine learning for edge devices.
The quality of any machine learning or deep learning model depends on the values that define the model structure and corresponding hyperparameters. Many practitioners may find themselves investing countless hours manually searching for the right model and related hyperparameter values. Some use highly inefficient grid search methods. Others will use simple random sampling, which actually works fairly well. But alone, this method only offers a globalized search, and other sampling methods may be better suited to the job.
Why not use machine learning to automate the search for the best model?
This presentation details an advanced approach that uses both global and local search strategies that can be evaluated in parallel to ensure a quick and efficient exploration of the decision space. In the case of this presentation, a genetic algorithm (GA) will be examined for the global search because the selection and crossover aspects of a GA distinguish it from a purely random search. A generating set search (GSS) will be used to greedily search the local decision space.
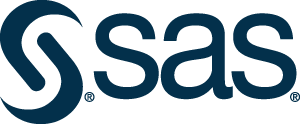
Each real-world application of machine learning owns a set of defining characteristics that distinguish it from other problems. In Nauto's case, we discovered that our driving data is remarkably uniform. By leveraging this trait, we were able to achieve massive optimizations in model accuracy and inference speed, directly improving Nauto's core safety offerings like Forward Collision Warning.
This talk will explore the importance of identifying domain-specific traits when deploying AI solutions to the world. It will show how leveraging the quirks of your problem can lead to optimizations that occasionally even contradict established conventions and common intuition.
Scanning Electron Microscope (SEM) images are the primary means used by expert engineers to identify and diagnose defects in semiconductor variable shape beam (VSB) mask writers. Deep learning (DL) offers an attractive alternative to this tedious process. However, extremely robust mask writers preclude collecting a large variety of SEM images to train DL models. Using digital twins that can mimic SEM images provides an exceptional way to synthesize ample DL training data. This talk will take a deep dive into synthesizing SEM images and leveraging them to build DL models for VSB mask writer defects analysis.
The increasing prevalence of high-fidelity industrial digital twins is providing a range of opportunities to apply Reinforcement Learning (RL) techniques outside of traditional academic examples and video games. While this trend is now well-established, most RL developments and deployments in the real world are done on an ad-hoc basis with little consideration given to how to repeat and scale similar initiatives in an efficient way. In this session we will address these shortcomings and illustrate them through our experience in optimising the design of a state-of-the-art sailing boat for a prominent competition with RL. Building an agent to control the boat is a very complex RL task for several reasons: imperfect information, loosely defined goals with delayed rewards, highly dynamic state and action spaces. In racing conditions, it takes a team of Olympic-level athletes to sail the boat and make it “fly” thanks to its underwater wings (read hydro-foiling). In order to control convergence variability and sampling efficiency, the working solution required a custom deep learning implementation of the Soft Actor Critic RL algorithm, with state of the art improvements such as experience replay buffer pooling, domain randomisation and curriculum learning. Beyond describing solutions to traditional RL considerations, we will also focus on the underlying workflows and technology stack required to carry out a deep learning project of this technical complexity in a scalable way. We will use facets of Software 2.0, such as higher-level APIs and the automation of end-to-end model development tasks, to highlight our iterative choices and the optimisation opportunities along the machine learning pipeline and ultimately the production system.
Join your fellow practitioners in this interactive session where you can exchange approaches to shared challenges and hear how your peers are tackling similar issues.
.
Deep Learning World - Virtual - Day 2 - Tuesday, May 25th, 2021
Grab your real coffee and share experiences virtually with your peers to explore the new challenges of operating in a largely virtual world. Just like pre-show breakfast in a regular conference you’ll join a “round table” with seven fellow attendees and see where the conversation takes you.
In the field of Speech Recognition, the state of the art for generic conversations has reached superhuman levels. However, things are not nearly as good in specialized knowledge domains: conversation in which people with accents speak in a noisy environment often results in high error rates. Considering the low performance of Speech Recognizers on real data, it becomes imperative to customize the end-to-end probabilistic model. This session will focus on discussing the fundamentals of speech recognition and how Cisco has moved from multiple phoneme-based models to a single end-to-end grapheme-based Recurrent Neural Network architecture that can transcribe audios directly while also reducing latency by tweaking the model during inference time.
Terms of service (ToS) violators at Stripe are merchants on our ecosystem who are selling items and services that are prohibited by our terms of service. This talk will present how we build a multimodal ToS violation deep learning detection system that combines text, images, and tabular data. We will also discuss how we enable interpretability of model predictions.
Image classification has been solved successfully for many tasks e.g. using deep learning techniques. However, in many application scenarios, the set of classes from which input images are drawn is not completely known at time of modeling. It is then important to a) reduce false positives during inference and b) enable the description of "unknown" image content during annotation. This case study shows how we addressed these issues at Miele for recognition of food items together with a concept for dealing with unkown classes during data annotation.
As online fraud increases in volume, malicious actors rely on automation to keep scaling. To spread false information, sell nonexistent products, or spend money from stolen credit cards, fraudsters use scripts and other automation tools to manage a large number of fake accounts. Such automation introduces a common thread in the profiles or communications of the accounts they control. We developed a deep learning model to detect suspicious patterns amongst different accounts. The model was successful in detecting coordinated attacks, even when deployed on a novel platform, increasing by up to 80% the detection of malicious actors.
Deep learning solutions are nowadays a standard tool in many technological fields. Specifically, in the microbiological field, this is possible through Full Laboratory Automations.
The combination of those two game-changers, made available by COPAN, allow microbiologists to streamline their daily routine: the preparation, incubation and evaluation of thousands of samples (mainly from negative analysis) focusing their high skilled qualities directly on the most challenging and critical ones.
In this talk, Giovanni Turra, key member of the Imaging and Data Analysis team at COPAN, explains how deep learning supports and empowers the daily laboratory battle against diseases.
Join your fellow practitioners in this interactive session where you can exchange approaches to shared challenges and hear how your peers are tackling similar issues.
.
Deep Learning World - Virtual - Day 3 - Wednesday, May 26th, 2021
Grab your real coffee and share experiences virtually with your peers to explore the new challenges of operating in a largely virtual world. Just like pre-show breakfast in a regular conference you’ll join a “round table” with seven fellow attendees and see where the conversation takes you.
Models generalize best when their complexity matches the problem. To avoid overfit, practitioners usually trade off accuracy with complexity, measured by the count of parameters. But this is surprisingly flawed. For example, a parameter is equivalent to one "degree of freedom" only for regression; it can be > 4 for decision trees, and < 1 for neural networks. Worse, a major source of complexity -- over-search — remains hidden. The vast exploration of potential model structures leaves no trace on the final (perhaps simple-looking) model, but has outsized influence over whether it is trustworthy.
I’ll show how Generalized Degrees of Freedom (GDF, by Ye) can be used to measure the full complexity of algorithmic modeling. This allows one to fairly compare very different models and be more confident about out-of-sample accuracy. GDF also makes clear how seemingly complex ensemble models avoid overfit, and lastly, reveals a new type of outlier -- cases having high model influence.
Deep learning models for forecasting and planning have shown significant promise for handling multiple variables, uncovering hidden patterns, and producing accurate forecasts. However, as one might expect, deep learning models are also complex and rife with pitfalls. Since these techniques often seem like a ‘black box,’ managers -- both technical and nontechnical backgrounds -- can find them hard to master.
In this session, Senior Data Scientist, Javed Ahmed will focus on the intuition behind various deep learning approaches, explore how managers can tackle highly complex models by asking the right questions, and evaluating the models with familiar tools.
Attendees at the Metis session will leave with the tools to:
● Identify types of forecasting applications that can benefit from deep learning
● Broadly understand deep learning approaches relevant to forecasting
● Understand pitfalls related to deep learning approaches, and why simpler models may work better
● Evaluate the results of a forecasting program
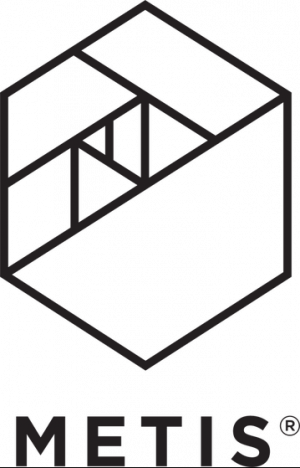
Graph embedding on very large graphs is a challenging task with many real-world applications such as fraud detection and cross-sell recommendation. The huge computational workload can only be efficiently handled by scalable distributed systems such as Spark or GPU-based deep learning frameworks such as PyTorch. Fugue is an open source Python framework which can manage various backend computing engines with constant interfaces. We will demonstrate how we scale up the transductive embedding algorithm “node2vec” using Spark to handle giant graphs in the Fugue framework. We also applied Fugue and Spark to the inductive embedding algorithm “GraphSAGE” for faster edge sampling.
Every year in Australia ~200 people lose their lives and 100,000+ are seriously injured in potentially avoidable work place incidents. The challenges presented by the extreme conditions and complex work environments typical in heavy industries need to be overcome. This talk presents an advanced computer vision edge AI/ML for these harsh environments. Details are presented on the journey from the R&D group of one of the world’s larger construction companies to a venture capital funded startup; along with the requisite non-trivial performance, market adoptability and technology scalability accomplishments, and the underpinning approaches for Computer Vision & Edge AI/ML.
Take a break or join your fellow practitioners in this interactive session where you can exchange approaches to shared challenges and hear how your peers are tackling similar issues.
Online fraud is flourishing as online services extend to more industries, including financial service providers, insurance companies, online retailers, and social networks. Network traffic data is invaluable for identifying such malicious actors. In this talk, we will show how to train a self-supervised deep learning model using only unlabeled network logs. Deriving the representation from the "structure" of the bits in an IP address, the model can process novel entities not encountered in the training data. Results show that our proposed framework can identify anomalous network accesses up to 16 times better than Amazon SageMaker's state-of-the-art model.
Since 2018, Transformer models revolutionized the planet NLP by bridging the gap between universal and task-specific representations. Transformers accelerated the shift from a world in which each NLP application requires training deep neural networks from scratch to the emergence of large pretrained models with great generalization capabilities, which are finetuned for downstream tasks with limited amount of labelled data. Yet, using Transformers for industrial applications poses various challenges. In this accessible presentation, Hadrien Van Lierde from Tencent-backed WeBank will describe how China’s leading digital bank uses Transformers to improve its customer service chatbot while maintaining low latency and high throughput.
Deep Learning World - Virtual - Day 4 - Thursday, May 27th, 2021
Grab your real coffee and share experiences virtually with your peers to explore the new challenges of operating in a largely virtual world. Just like pre-show breakfast in a regular conference you’ll join a “round table” with seven fellow attendees and see where the conversation takes you.
Deep learning and Computer Vision are changing the way to improve product quality in manufacturing industry. We have used cutting edge neural network architectures to identify the source of problems in products. It improves the product quality by improved defect detection, defect categorization and enhances the customer experience. The models are deployed into production and are generating fantastic results. It will be surely a great attraction for the visitors who want to analyze the significance of deep learning, identify the process and challenges. It will be a first hand information for them which will prove to be really useful and will have far-reaching results.
Join your fellow practitioners in this interactive session where you can exchange approaches to shared challenges and hear how your peers are tackling similar issues.
For machine learning problems, we don't lack great tools for certain problems, we lack a unified approach to use them for both prototyping and production. Fugue is a framework, aiming to bridge this gap. In this talk, we are going through a real deep learning example with preprocessing, training, and hyperparameter tuning. We will discuss the pain points and demonstrate how you can use Fugue to quickly iterate on small data and scale up on a Spark cluster without code change. We may also talk about some Lyft use cases, and how Fugue changed the game.
Take a break or join your fellow practitioners in this interactive session where you can exchange approaches to shared challenges and hear how your peers are tackling similar issues.
Many organizations deal with incoming customer problems either internally or externally. Frequently, these problems are handled one at a time by humans. We’ll discuss how at Google we are doing early detection of broad IT incidents before they become too large. We'll go through unsupervised learning techniques that when combined with deep learning-based language modeling creates a powerful, robust system that has saved Googlers hundreds of thousands of hours in productivity.
Thomson Reuters produces timely financial news and alerts that is used by many of our corporate and institutional customers. This is extracted from multiple sources, including corporate disclosures, and presented as an ongoing series of alerts and bulletins. One of the major challenges in this kind of financial work is the lack of accurate or weak training data which required us to employ various innovative data cleaning techniques. We also discuss our NLP-based production system that makes strong use of a BERT pre-trained model in combination with an unsupervised strategy, as well as some of the engineering challenges around making the system operate adequately in real-time.
Deep Learning World - Virtual - Day 5 - Friday, May 28th, 2021
Deep Learning image classifiers represent a breakthrough in image recognition and classification tasks. However they come with their own quirks and kinks: A "clean" product image can be easily classified, but what about a stock image of "a woman running in the rain"? Is it a raincoat? Her shoes? The phone cover? At Shopify we were tasked with mapping a large, complex, and "dirty" catalogue of products into duplicates, similar products and categories. In this case study I will walk you through our journey and how we were able to harness the strengths of CNNs while avoiding the major pitfalls.
Join your fellow practitioners in this interactive session where you can exchange approaches to shared challenges and hear how your peers are tackling similar issues.
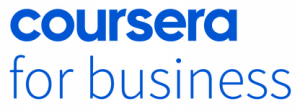
In today's world of elastic cloud/on-prem resources to support deployments, it is essential to be right-sized. We present a Hybrid Deep Learning and Statistical approach to model future demand. An accurate measure of incoming demand enables us to be right-sized while keeping guarantees on reliability, resiliency, and availability. We will share our experience dealing with demand volatility and how we temper them to enable actionability with tradeoffs.
Take a break or join your fellow practitioners in this interactive session where you can exchange approaches to shared challenges and hear how your peers are tackling similar issues.
Combining automated speech recognition with natural language processing allows one to build semi-automatic note taking apps. With a minimal input from the call participants, it would create transcribe speech on the fly and extract and highlight intents and entities, thus creating a semi-structured meeting minutes, ready to be exported to structured databases. For practical applications, it is important for the system to recognize custom lexicon, without expensive acoustic model retraining, solely by automatically building custom language models.
The number of Earth observation satellites has increased significantly and these satellites are producing huge volumes of images at different spatial resolutions and spectral bands. Manually understanding all the pixels is nearly impossible, as it would take years simply to look at them. This talk will cover the challenges and approaches involved in understanding satellite imagery, which are different than those involved in working with other real-world images. At Orbital Insight, we transform multiple sources of geospatial data - including satellite images - into actionable insights to understand economic, societal, and environmental trends at global, regional, and hyper-local scales.